LangChain
什么是 LangChain
?
LangChain 并不完美,还在不断迭代中,学习 LangChain 更多的是借鉴其思想,具体的接口和模块可能很快就会改变。
LangChain 的核心组件
模型 I/O 封装
LLMs
:大语言模型Chat Models
:一般基于 LLMs,但按对话结构重新封装PromptTemple
:提示词模板OutputParser
:解析输出
数据连接封装
Document Loaders
:各种格式文件的加载器Document Transformers
:对文档的常用操作,如:split, filter, translate, extract metadata, etcText Embedding Models
:文本向量化表示,用于检索等操作(啥意思?别急,后面详细讲)Verctorstores
: (面向检索的)向量的存储Retrievers
: 向量的检索
记忆封装
Memory
:这里不是物理内存,从文本的角度,可以理解为“上文”、“历史记录”或者说“记忆力”的管理
架构封装
Chain
:实现一个功能或者一系列顺序功能组合Agent
:根据用户输入,自动规划执行步骤,自动选择每步需要的工具,最终完成用户指定的功能Tools
:调用外部功能的函数,例如:调 google 搜索、文件 I/O、Linux Shell 等等Toolkits
:操作某软件的一组工具集,例如:操作 DB、操作 Gmail 等等
Callbacks 封装
Callbacks
:在 Agent 的执行过程中,可以插入一些回调函数,用于实现一些特殊的功能,例如:记录日志、调用外部服务、调用外部工具等等。
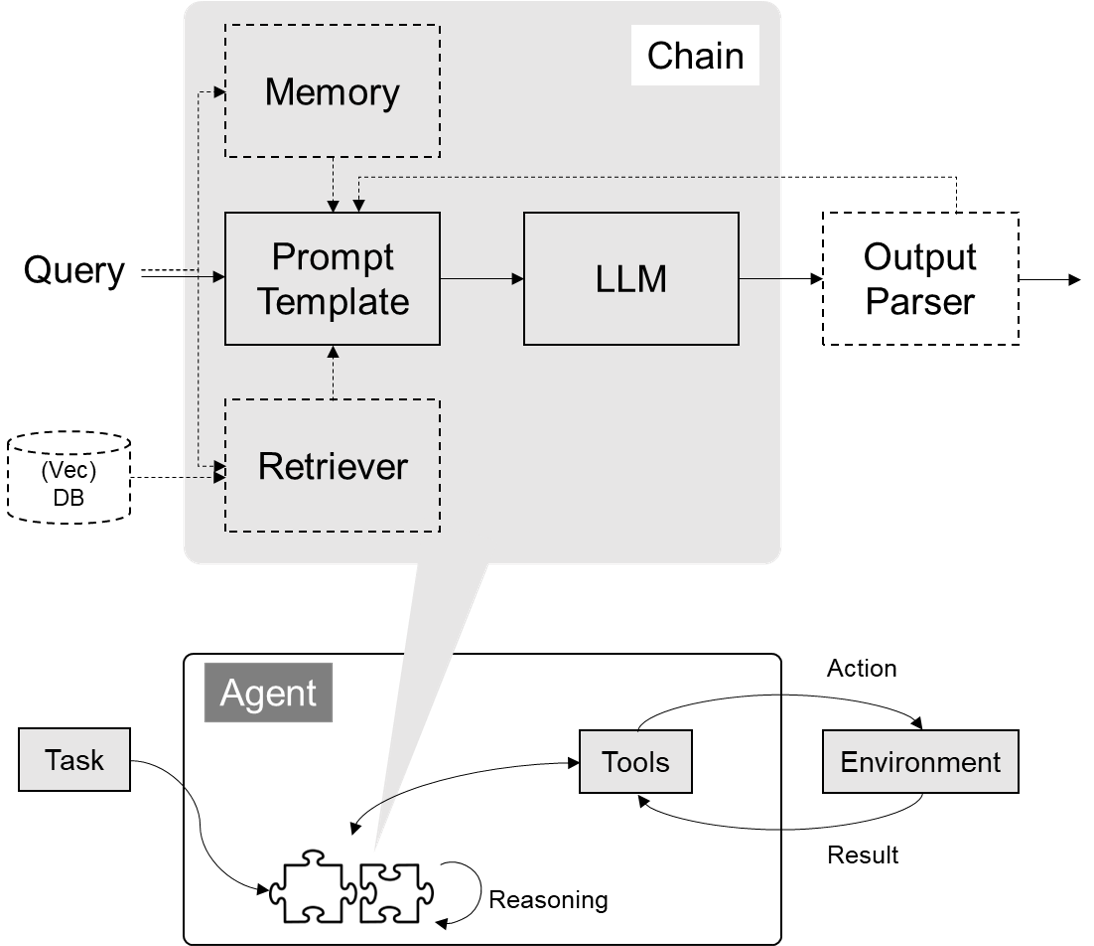
模型 I/O
封装
模型 API:LLM vs. ChatModel
# 安装最新版本 !pip install langchain==0.0.292
1)生成模型封装
from langchain.llms import OpenAI from langchain.chat_models import ChatOpenAI llm = OpenAI() # 默认是text-davinci-003模型 llm.predict("你好,欢迎")
'您访问我们的网站。我们提供了丰富的产品,包括家具、家居用品、家用电器、服装和鞋子等等,还有定制服务,以满足您的不同需求。我们的售后服务质量也很高,您可以随时联系我们,如果您有任何问题,我们都会竭诚为您服务。'
2)对话模型封装
chat_model = ChatOpenAI() # 默认是gpt-3.5-turbo chat_model.predict("你好,欢迎")
3)多轮对话 Session 封装
from langchain.schema import ( AIMessage, # 等价于OpenAI接口中的assistant role HumanMessage, # 等价于OpenAI接口中的user role SystemMessage # 等价于OpenAI接口中的system role ) messages = [ SystemMessage(content="你是 AGIClass 的课程助理。"), HumanMessage(content="我来上课了") ] chat_model(messages)
AIMessage(content='欢迎上课!请问你是哪位学生?', additional_kwargs={}, example=False)
不同模型统一接口调用
from langchain.chat_models import ErnieBotChat from langchain.schema import HumanMessage chat_model = ErnieBotChat() messages = [ HumanMessage(content="你是谁") ] chat_model(messages)
AIMessage(content='我是百度公司开发的文心一言,英文名是ERNIE Bot,可以协助您完成范围广泛的任务并提供有关各种主题的信息,比如回答问题,提供定义和解释及建议。如果您有任何问题,请随时向我提问。', additional_kwargs={}, example=False)
模型的输入与输出
1)Prompt 模板封装
PromptTemplate
from langchain.prompts import PromptTemplate template = PromptTemplate.from_template("给我讲个关于{subject}的笑话") print(template.input_variables) print(template.format(subject='小明'))
['subject'] 给我讲个关于小明的笑话
ChatPromptTemplate
from langchain.prompts import ChatPromptTemplate from langchain.prompts.chat import SystemMessagePromptTemplate, HumanMessagePromptTemplate from langchain.chat_models import ChatOpenAI template = ChatPromptTemplate.from_messages( [ SystemMessagePromptTemplate.from_template("你是{product}的客服助手。你的名字叫{name}"), HumanMessagePromptTemplate.from_template("{query}"), ] ) llm = ChatOpenAI() llm( template.format_messages( product="AGI课堂", name="瓜瓜", query="你是谁" ) )
AIMessage(content='我是瓜瓜,AGI课堂的客服助手。有什么我可以帮助你的吗?', additional_kwargs={}, example=False)
提示:把 Prompt 模板看作带有参数的函数,下面的内容可能更好理解
FewShotPromptTemplate
from langchain.prompts.few_shot import FewShotPromptTemplate from langchain.prompts import PromptTemplate #例子(few-shot) examples = [ { "input": "北京天气怎么样", "output" : "北京市" }, { "input": "南京下雨吗", "output" : "南京市" }, { "input": "江城热吗", "output" : "武汉市" } ] #例子拼装的格式 example_prompt = PromptTemplate(input_variables=["input", "output"], template="Input: {input}\nOutput: {output}") #Prompt模板 prompt = FewShotPromptTemplate( examples=examples, example_prompt=example_prompt, suffix="Input: {input}\nOutput:", input_variables=["input"] ) prompt = prompt.format(input="羊城多少度") print("===Prompt===") print(prompt) llm = OpenAI() response = llm(prompt) print("===Response===") print(response)
===Prompt=== Input: 北京天气怎么样 Output: 北京市 Input: 南京下雨吗 Output: 南京市 Input: 江城热吗 Output: 武汉市 Input: 羊城多少度 Output: ===Response=== 广州市
OutputParser
1)Pydantic (JSON) Parser
自动根据 Pydantic 类的定义,生成输出的格式说明。
from langchain.prompts import PromptTemplate, ChatPromptTemplate, HumanMessagePromptTemplate from langchain.llms import OpenAI from langchain.output_parsers import PydanticOutputParser from pydantic import BaseModel, Field, validator from typing import List, Dict import json # 避免print时中文变成unicode码 def chinese_friendly(string): lines = string.split('\n') for i, line in enumerate(lines): if line.startswith('{') and line.endswith('}'): try: lines[i] = json.dumps(json.loads(line), ensure_ascii=False) except: pass return '\n'.join(lines) model_name = 'gpt-4' temperature = 0 model = OpenAI(model_name=model_name, temperature=temperature) # 定义你的输出格式 class Command(BaseModel): command: str = Field(description="linux shell命令名") arguments: Dict[str, str] = Field(description="命令的参数 (name:value)") # 你可以添加自定义的校验机制 @validator('command') def no_space(cls, field): if " " in field or "\t" in field or "\n" in field: raise ValueError("命令名中不能包含空格或回车!") return field # 根据Pydantic对象的定义,构造一个OutputParser parser = PydanticOutputParser(pydantic_object=Command) prompt = PromptTemplate( template="将用户的指令转换成linux命令.\n{format_instructions}\n{query}", input_variables=["query"], # 直接从OutputParser中获取输出描述,并对模板的变量预先赋值 partial_variables={"format_instructions": parser.get_format_instructions()} ) print("====Format Instruction=====") print(chinese_friendly(parser.get_format_instructions())) query = "将系统日期设为2023-04-01" model_input = prompt.format_prompt(query=query) print("====Prompt=====") print(chinese_friendly(model_input.to_string())) output = model(model_input.to_string()) print("====Output=====") print(output) print("====Parsed=====") cmd = parser.parse(output) print(cmd)
/*/opt/conda/lib/python3.11/site-packages/langchain/llms/openai.py:200: UserWarning: You are trying to use a chat model. This way of initializing it is no longer supported. Instead, please use: `from langchain.chat_models import ChatOpenAI` warnings.warn( /opt/conda/lib/python3.11/site-packages/langchain/llms/openai.py:787: UserWarning: You are trying to use a chat model. This way of initializing it is no longer supported. Instead, please use: `from langchain.chat_models import ChatOpenAI` warnings.warn(*/ ====Format Instruction===== The output should be formatted as a JSON instance that conforms to the JSON schema below. As an example, for the schema {"properties": {"foo": {"title": "Foo", "description": "a list of strings", "type": "array", "items": {"type": "string"}}}, "required": ["foo"]} the object {"foo": ["bar", "baz"]} is a well-formatted instance of the schema. The object {"properties": {"foo": ["bar", "baz"]}} is not well-formatted. Here is the output schema: ``` {"properties": {"command": {"title": "Command", "description": "linux shell命令名", "type": "string"}, "arguments": {"title": "Arguments", "description": "命令的参数 (name:value)", "type": "object", "additionalProperties": {"type": "string"}}}, "required": ["command", "arguments"]} ``` ====Prompt===== 将用户的指令转换成linux命令. The output should be formatted as a JSON instance that conforms to the JSON schema below. As an example, for the schema {"properties": {"foo": {"title": "Foo", "description": "a list of strings", "type": "array", "items": {"type": "string"}}}, "required": ["foo"]} the object {"foo": ["bar", "baz"]} is a well-formatted instance of the schema. The object {"properties": {"foo": ["bar", "baz"]}} is not well-formatted. Here is the output schema: ``` {"properties": {"command": {"title": "Command", "description": "linux shell命令名", "type": "string"}, "arguments": {"title": "Arguments", "description": "命令的参数 (name:value)", "type": "object", "additionalProperties": {"type": "string"}}}, "required": ["command", "arguments"]} ``` 将系统日期设为2023-04-01 ====Output===== { "command": "date", "arguments": { "-s": "2023-04-01" } } ====Parsed===== command='date' arguments={'-s': '2023-04-01'}
2)Auto-Fixing Parser
利用LLM自动根据解析异常修复并重新解析
from langchain.output_parsers import OutputFixingParser new_parser = OutputFixingParser.from_llm(parser=parser, llm=ChatOpenAI(model="gpt-4")) #我们把之前output的格式改错 output = output.replace("\"","'") print("===格式错误的Output===") print(output) try: cmd = parser.parse(output) except Exception as e: print("===出现异常===") print(e) #用OutputFixingParser自动修复并解析 cmd = new_parser.parse(output) print("===重新解析结果===") print(cmd)
===格式错误的Output=== { 'command': 'date', 'arguments': { '-s': '2023-04-01' } } ===出现异常=== Failed to parse Command from completion { 'command': 'date', 'arguments': { '-s': '2023-04-01' } }. Got: Expecting property name enclosed in double quotes: line 2 column 3 (char 4) ===重新解析结果=== command='date' arguments={'-s': '2023-04-01'}
数据连接封装
文档加载器:Document Loaders
llama2
的论文作为文档文件。
!pip install pypdf
from langchain.document_loaders import PyPDFLoader loader = PyPDFLoader("llama2.pdf") pages = loader.load_and_split() print(pages[0].page_content)
Llama 2 : Open Foundation and Fine-Tuned Chat Models Hugo Touvron∗Louis Martin†Kevin Stone† Peter Albert Amjad Almahairi Yasmine Babaei Nikolay Bashlykov Soumya Batra Prajjwal Bhargava Shruti Bhosale Dan Bikel Lukas Blecher Cristian Canton Ferrer Moya Chen Guillem Cucurull David Esiobu Jude Fernandes Jeremy Fu Wenyin Fu Brian Fuller Cynthia Gao Vedanuj Goswami Naman Goyal Anthony Hartshorn Saghar Hosseini Rui Hou Hakan Inan Marcin Kardas Viktor Kerkez Madian Khabsa Isabel Kloumann Artem Korenev Punit Singh Koura Marie-Anne Lachaux Thibaut Lavril Jenya Lee Diana Liskovich Yinghai Lu Yuning Mao Xavier Martinet Todor Mihaylov Pushkar Mishra Igor Molybog Yixin Nie Andrew Poulton Jeremy Reizenstein Rashi Rungta Kalyan Saladi Alan Schelten Ruan Silva Eric Michael Smith Ranjan Subramanian Xiaoqing Ellen Tan Binh Tang Ross Taylor Adina Williams Jian Xiang Kuan Puxin Xu Zheng Yan Iliyan Zarov Yuchen Zhang Angela Fan Melanie Kambadur Sharan Narang Aurelien Rodriguez Robert Stojnic Sergey Edunov Thomas Scialom∗ GenAI, Meta Abstract In this work, we develop and release Llama 2, a collection of pretrained and fine-tuned large language models (LLMs) ranging in scale from 7 billion to 70 billion parameters. Our fine-tuned LLMs, called Llama 2-Chat , are optimized for dialogue use cases. Our models outperform open-source chat models on most benchmarks we tested, and based on ourhumanevaluationsforhelpfulnessandsafety,maybeasuitablesubstituteforclosed- source models. We provide a detailed description of our approach to fine-tuning and safety improvements of Llama 2-Chat in order to enable the community to build on our work and contribute to the responsible development of LLMs. ∗Equal contribution, corresponding authors: {tscialom, htouvron}@meta.com †Second author Contributions for all the authors can be found in Section A.1.arXiv:2307.09288v2 [cs.CL] 19 Jul 2023
文档处理器
1)TextSplitter
import re, wordninja #预处理字符全都连在一起的行 def preprocess(text): def split(line): tokens = re.findall(r'\w+|[.,!?;%$-+=@#*/]', line) return [ ' '.join(wordninja.split(token)) if token.isalnum() else token for token in tokens ] lines = text.split('\n') for i,line in enumerate(lines): if len(max(line.split(' '), key = len)) >= 20: lines[i] = ' '.join(split(line)) return ' '.join(lines)
from langchain.text_splitter import RecursiveCharacterTextSplitter text_splitter = RecursiveCharacterTextSplitter( chunk_size=200, chunk_overlap=50, # 思考:为什么要做overlap length_function=len, add_start_index=True, ) paragraphs = text_splitter.create_documents([preprocess(pages[3].page_content)]) for para in paragraphs: print(para.page_content) print('-------')
Figure 3: Safety human evaluation results for Llama 2-Chat compared to other open-source and closed- source models. Human raters judged model generations for safety violations across ~2,000 ------- generations for safety violations across ~2,000 adversarial prompts consisting of both single and multi-turn prompts. More details can be found in Section 4.4. It is important to caveat these safety ------- 4.4. It is important to caveat these safety results with the inherent bias of LL M evaluations due to limitations of the prompt set , subjectivity of the review guidelines , and subjectivity of ------- of the review guidelines , and subjectivity of individual rate rs . Additionally , these safety evaluations are performed using content standards that are likely to be biased towards the Llama ------- that are likely to be biased towards the Llama 2-Chatmodels. We are releasing the following models to the general public for research and commercial use‡: 1 . Llama 2 , an updated version of Llama 1 ------- use‡: 1 . Llama 2 , an updated version of Llama 1 , trained on a new mix of publicly available data . We also increased the size of the pre training corpus by 40 % , doubled the context length of the ------- by 40 % , doubled the context length of the model , and adopted grouped query attention ( A in s lie et al . , 2023 ) . We are releasing variants of Llama 2 with 7 B , 13 B , and 70 B parameters . We ------- 2 with 7 B , 13 B , and 70 B parameters . We have also trained 34 B variants , which were port on in this paper but are not releasing.§ 2.Llama 2-Chat , a fine-tuned version of Llama 2 that is ------- 2-Chat , a fine-tuned version of Llama 2 that is optimized for dialogue use cases. We release variants of this model with 7B, 13B, and 70B parameters as well. We believe that the open release of LL ------- as well. We believe that the open release of LL Ms , when done safely , will be a net benefit to society . Like all LL Ms , Llama 2 is a new technology that carries potential risks with use (Bender ------- that carries potential risks with use (Bender et al., 2021b; Weidinger et al., 2021; Sola i manet al . , 2023 ) . Testing conducted to date has been in English and has not and could not cover all ------- in English and has not and could not cover all scenarios. Therefore, before deploying any applications of Llama 2-Chat , developers should perform safety testing and tuning tailored to their specific ------- testing and tuning tailored to their specific applications of the model . We provide a responsible use guide¶and code examples‖to facilitate the safe deployment of Llama 2 andLlama 2-Chat . More ------- safe deployment of Llama 2 andLlama 2-Chat . More details of our responsible release strategy can be found in Section 5.3. The remainder of this paper describes our pre training methodology ( Section ------- describes our pre training methodology ( Section 2 ) , fine tuning methodology (Section 3), approach to model safety (Section 4), key observations and insights (Section 5), relevant related work ------- and insights (Section 5), relevant related work (Section 6), and conclusions (Section 7). https / / a i . meta . com / resources / models and libraries / llama / §We are delaying the release of the ------- / llama / §We are delaying the release of the 34B model due to a lack of time to sufficiently red team. https / / a i . meta . com / llama https / / g it hub . com / facebook research / llama 4 -------
2)Doctran
!pip install doctran
from langchain.document_transformers import DoctranTextTranslator translator = DoctranTextTranslator( openai_api_model="gpt-3.5-turbo", language="Chinese" ) translated_document = await translator.atransform_documents([pages[3]]) print(translated_document[0].page_content)
图3:Llama 2-Chat与其他开源和闭源模型的安全人工评估结果。人工评估员对大约2000个对抗性提示进行了安全违规的模型生成评判,包括单轮和多轮提示。更多细节请参见第4.4节。需要注意的是,由于提示集的限制、审查指南的主观性和个体评估员的主观性,这些安全评估结果可能存在固有的LLM评估偏差。此外,这些安全评估是使用可能对Llama 2-Chat模型有偏见的内容标准进行的。我们向公众发布以下模型供研究和商业用途‡:1. Llama 2,Llama 1的更新版本,使用新的公开可用数据进行训练。我们还将预训练语料库的大小增加了40%,将模型的上下文长度加倍,并采用了分组查询注意力(Ainslie等,2023)。我们发布了7B、13B和70B参数的Llama 2变体。我们还训练了34B变体,在本文中进行了报告,但不发布§。2. Llama 2-Chat,Llama 2的经过微调的版本,针对对话使用案例进行了优化。我们发布了7B、13B和70B参数的Llama 2-Chat变体。我们相信,安全地公开LLM将对社会产生净利益。像所有LLM一样,Llama 2是一项新技术,使用时存在潜在风险(Bender等,2021b; Weidinger等,2021; Solaiman等,2023)。迄今为止进行的测试是用英语进行的,无法涵盖所有场景。因此,在部署Llama 2-Chat的任何应用程序之前,开发人员应根据其特定的模型应用进行安全测试和调优。我们提供了一个负责任的使用指南¶和代码示例‖,以促进Llama 2和Llama 2-Chat的安全部署。有关我们负责任发布策略的更多细节,请参见第5.3节。本文的其余部分描述了我们的预训练方法(第2节),微调方法(第3节),模型安全方法(第4节),关键观察和见解(第5节),相关工作(第6节)和结论(第7节)。‡https://ai.meta.com/resources/models-and-libraries/llama/§由于缺乏足够的时间进行充分的红队测试,我们推迟了34B模型的发布。¶https://ai.meta.com/llama‖https://github.com/facebookresearch/llama
检索与问答
from langchain.retrievers import TFIDFRetriever # 最传统的关键字加权检索 from langchain.text_splitter import RecursiveCharacterTextSplitter import wordninja, re text_splitter = RecursiveCharacterTextSplitter( chunk_size=200, chunk_overlap=60, length_function=len, add_start_index=True, ) # 取一个有信息量的章节(Introduction: 第2-3页) paragraphs = text_splitter.create_documents( [preprocess(d.page_content) for d in pages[2:4]] ) user_query = "Does llama 2 have a dialogue version?" retriever = TFIDFRetriever.from_documents(paragraphs) docs = retriever.get_relevant_documents(user_query) print(docs[0].page_content)
34 B variants , which were port on in this paper but are not releasing.§ 2.Llama 2-Chat , a fine-tuned version of Llama 2 that is optimized for dialogue use cases. We release variants of this model
这里,暂时先手写一个问答过程
from langchain.prompts import ChatPromptTemplate from langchain.prompts.chat import SystemMessagePromptTemplate, HumanMessagePromptTemplate from langchain.chat_models import ChatOpenAI template = ChatPromptTemplate.from_messages( [ SystemMessagePromptTemplate.from_template( "你是问答机器人,你根据以下信息回答用户问题。\n" + "已知信息:\n{information}\n\nBe brief, and do not make up information."), HumanMessagePromptTemplate.from_template("{query}"), ] ) llm = ChatOpenAI(temperature=0) response = llm( template.format_messages( information=docs[0].page_content, query=user_query ) ) print(response.content)
Yes, Llama 2 has a dialogue version called Llama 2-Chat. It is a fine-tuned version of Llama 2 that is optimized for dialogue use cases.
# 换个问法 user_query = "Does llama 2 have a conversational variant?" retriever = TFIDFRetriever.from_documents(paragraphs) docs = retriever.get_relevant_documents(user_query) print("===检索结果===") print(docs[0].page_content) response = llm( template.format_messages( information=docs[0].page_content, query=user_query ) ) print("===回答===") print(response.content)
===检索结果=== is simple , high computational requirements have limited the development of LLMs to a few players. There have been public releases of pretrained LLMs (such as BLOOM (Scao et al., 2022), LLaMa-1 ===回答=== There is no information available about a conversational variant of LLaMa-2.
from langchain.embeddings.openai import OpenAIEmbeddings from langchain.vectorstores import FAISS embeddings = OpenAIEmbeddings() db = FAISS.from_documents(paragraphs, embeddings) #Facebook的开源向量检索引擎 user_query = "Does llama 2 have a conversational variant?" docs = db.similarity_search(user_query) print("===检索结果===") print(docs[0].page_content) response = llm( template.format_messages( information=docs[0].page_content, query=user_query ) ) print("===回答===") print(response.content)
===检索结果=== 34 B variants , which were port on in this paper but are not releasing.§ 2.Llama 2-Chat , a fine-tuned version of Llama 2 that is optimized for dialogue use cases. We release variants of this model ===回答=== Yes, Llama 2 has a conversational variant called Llama 2-Chat. It is a fine-tuned version of Llama 2 that is optimized for dialogue use cases.
尝试跨语言检索
user_query = "llama 2有对话式的版本吗" docs = db.similarity_search(user_query) print("===检索结果===") print(docs[0].page_content) response = llm( template.format_messages( information=docs[0].page_content, query=user_query ) ) print("===回答===") print(response.content)
===检索结果=== 34 B variants , which were port on in this paper but are not releasing.§ 2.Llama 2-Chat , a fine-tuned version of Llama 2 that is optimized for dialogue use cases. We release variants of this model ===回答=== 是的,Llama 2有一个专门针对对话场景进行优化的版本,称为Llama 2-Chat。
文档向量化:Text Embeddings
Embedding
:将目标物体(词、句子、文章)表示成向量的方法
from langchain.embeddings import OpenAIEmbeddings embeddings = OpenAIEmbeddings() # 默认是text-embedding-ada-002 text = "这是一个测试" document = "测试文档" query_vec = embeddings.embed_query(text) doc_vec = embeddings.embed_documents([document]) print(len(query_vec)) print(query_vec[:10]) # 为了展示方便,只打印前10维 print(len(doc_vec[0])) print(doc_vec[0][:10]) # 为了展示方便,只打印前10维
1536 [-0.011436891812873518, -0.012987656599242918, 0.009020282942780396, -0.011973197646077209, -0.02477347121082455, 0.014486729257110757, -0.02189163318421738, -0.005188601123634472, -0.0012567658055167737, -0.0337032938600498] 1536 [-0.002583218747341917, 0.0008178391754881199, -0.0045899870032351945, -0.005638406631416479, -0.010556249915691514, 0.02594027304651135, -0.014526552570770228, -0.002125661361839841, -0.01431758986133824, -0.018316714156232938]
向量的存储与索引:Vectorstores
from langchain.embeddings.openai import OpenAIEmbeddings from langchain.vectorstores import FAISS embeddings = OpenAIEmbeddings() db = FAISS.from_documents(paragraphs, embeddings) user_query = "where are the training data for llama 2 from" docs = db.similarity_search(user_query) print(docs[0].page_content) #response = llm(template.format_messages(information=docs[0].page_content,query=user_query)) #print(f"===回答===\n{response.content}")
to the general public for research and commercial use‡: 1 . Llama 2 , an updated version of Llama 1 , trained on a new mix of publicly available data . We also increased the size of the pre training
向量数据库功能对比
Name | Web GUI | GPU Support | Remote Support(HTTP/gRPC) | Cloud Native | Opensource | Metadata(hybrid search) |
---|---|---|---|---|---|---|
FAISS | N | Y | N | N | Y | N |
Pinecone | Y | N/A | Y | Y | N | Y |
Milvus | Y | Y | Y | Y | Y | Y |
Weaviate | N | N | Y | Y | Y | Y |
Qdrant | N | Y | Y | Y | Y | Y |
PGVector | N | N | Y | Y | Y | Y |
RediSearch | N | N | Y | Y | Y | Y |
向量检索:Retrievers
retriever = db.as_retriever() docs = retriever.get_relevant_documents(user_query) print(docs[0].page_content)
to the general public for research and commercial use‡: 1 . Llama 2 , an updated version of Llama 1 , trained on a new mix of publicly available data . We also increased the size of the pre training
1)Parent Document Retriever
从相关段落召回整个文档
from langchain.retrievers import ParentDocumentRetriever from langchain.text_splitter import RecursiveCharacterTextSplitter from langchain.storage import InMemoryStore from langchain.docstore import InMemoryDocstore from langchain.docstore.document import Document import faiss text_splitter = RecursiveCharacterTextSplitter( chunk_size=200, chunk_overlap=60, length_function=len, add_start_index=True, ) embedding_size = 1536 # OpenAIEmbeddings的维度 index = faiss.IndexFlatL2(embedding_size) # 精准检索 embedding_fn = OpenAIEmbeddings().embed_query # 构造向量数据库 vectorstore = FAISS(embedding_fn, index, InMemoryDocstore({}), {}) # 文档存储 store = InMemoryStore() retriever = ParentDocumentRetriever( vectorstore=vectorstore, docstore=store, child_splitter=text_splitter, ) retriever.add_documents(pages[:4], ids=None) user_query = "can llama2 be used for commercial purposes?" sub_docs = vectorstore.similarity_search(user_query) print("===段落===") print(sub_docs[0].page_content) retrieved_docs = retriever.get_relevant_documents(user_query) print("===文档===") print(retrieved_docs[0].page_content)
===段落=== 2-Chatmodels. We are releasing the following models to the general public for research and commercial use‡: 1.Llama 2 ,anupdatedversionof Llama 1,trainedonanewmixofpubliclyavailabledata. Wealso ===文档=== Figure 3: Safety human evaluation results for Llama 2-Chat compared to other open-source and closed- source models. Human raters judged model generations for safety violations across ~2,000 adversarial prompts consisting of both single and multi-turn prompts. More details can be found in Section 4.4. It is importanttocaveatthesesafetyresultswiththeinherentbiasofLLMevaluationsduetolimitationsofthe promptset,subjectivityofthereviewguidelines,andsubjectivityofindividualraters. Additionally,these safety evaluations are performed using content standards that are likely to be biased towards the Llama 2-Chatmodels. We are releasing the following models to the general public for research and commercial use‡: 1.Llama 2 ,anupdatedversionof Llama 1,trainedonanewmixofpubliclyavailabledata. Wealso increasedthesizeofthepretrainingcorpusby40%,doubledthecontextlengthofthemodel,and adoptedgrouped-queryattention(Ainslieetal.,2023). Wearereleasingvariantsof Llama 2 with 7B,13B,and70Bparameters. Wehavealsotrained34Bvariants,whichwereportoninthispaper but are not releasing.§ 2.Llama 2-Chat , a fine-tuned version of Llama 2 that is optimized for dialogue use cases. We release variants of this model with 7B, 13B, and 70B parameters as well. WebelievethattheopenreleaseofLLMs,whendonesafely,willbeanetbenefittosociety. LikeallLLMs, Llama 2 is a new technology that carries potential risks with use (Bender et al., 2021b; Weidinger et al., 2021; Solaimanet al.,2023). Testingconductedtodate hasbeeninEnglish andhasnot— andcouldnot— cover all scenarios. Therefore, before deploying any applications of Llama 2-Chat , developers should perform safetytestingand tuningtailoredtotheirspecificapplicationsofthemodel. Weprovidearesponsibleuse guide¶and code examples‖to facilitate the safe deployment of Llama 2 andLlama 2-Chat . More details of our responsible release strategy can be found in Section 5.3. Theremainderofthispaperdescribesourpretrainingmethodology(Section2),fine-tuningmethodology (Section 3), approach to model safety (Section 4), key observations and insights (Section 5), relevant related work (Section 6), and conclusions (Section 7). ‡https://ai.meta.com/resources/models-and-libraries/llama/ §We are delaying the release of the 34B model due to a lack of time to sufficiently red team. ¶https://ai.meta.com/llama ‖https://github.com/facebookresearch/llama 4
2)Reranker(可选)
LangChain 里目前没有,但是实际生产中会碰到需要 rerank 的情况!
支持Retrieval+Rerank的开源框架,参考:https://docs.jina.ai/
LangChain 自带的机制:
记忆封装:Memory
对话上下文:ConversationBufferMemory
from langchain.memory import ConversationBufferMemory, ConversationBufferWindowMemory history = ConversationBufferMemory() history.save_context({"input": "你好啊"}, {"output": "你也好啊"}) print(history.load_memory_variables({})) history.save_context({"input": "你再好啊"}, {"output": "你又好啊"}) print(history.load_memory_variables({}))
{'history': 'Human: 你好啊\nAI: 你也好啊'} {'history': 'Human: 你好啊\nAI: 你也好啊\nHuman: 你再好啊\nAI: 你又好啊'}
Message格式
from langchain.memory import ChatMessageHistory history = ChatMessageHistory() history.add_user_message("你好!") history.add_ai_message("有什么可以帮您?") print(history)
messages=[HumanMessage(content='你好!', additional_kwargs={}, example=False), AIMessage(content='有什么可以帮您?', additional_kwargs={}, example=False)]
只保留一个窗口的上下文
from langchain.memory import ConversationBufferWindowMemory window = ConversationBufferWindowMemory(k=2) window.save_context({"input": "第一轮问"}, {"output": "第一轮答"}) window.save_context({"input": "第二轮问"}, {"output": "第二轮答"}) window.save_context({"input": "第三轮问"}, {"output": "第三轮答"}) print(window.load_memory_variables({}))
{'history': 'Human: 第二轮问\nAI: 第二轮答\nHuman: 第三轮问\nAI: 第三轮答'}
自动对历史信息做摘要:ConversationSummaryMemory
from langchain.memory import ConversationSummaryMemory from langchain.llms import OpenAI memory = ConversationSummaryMemory( llm=OpenAI(temperature=0), # buffer="The conversation is between a customer and a sales." buffer="以中文表示" ) memory.save_context( {"input": "你好"}, {"output": "你好,我是你的AI助手。我能为你回答有关 AGIClass 的各种问题。"}) print(memory.load_memory_variables({}))
{'history': '\n人类问AI助手你好,AI助手回答你好,表示自己是人类的AI助手,可以回答有关AGIClass的各种问题。'}
用向量数据库存储记忆
from datetime import datetime from langchain.embeddings.openai import OpenAIEmbeddings from langchain.llms import OpenAI from langchain.memory import VectorStoreRetrieverMemory from langchain.chains import ConversationChain from langchain.prompts import PromptTemplate import faiss from langchain.docstore import InMemoryDocstore from langchain.vectorstores import FAISS embedding_size = 1536 # OpenAIEmbeddings的维度 index = faiss.IndexFlatL2(embedding_size) embedding_fn = OpenAIEmbeddings().embed_query vectorstore = FAISS(embedding_fn, index, InMemoryDocstore({}), {}) # 实际应用中k可以稍大一些,这里k=1演示方便 retriever = vectorstore.as_retriever(search_kwargs=dict(k=1)) memory = VectorStoreRetrieverMemory(retriever=retriever) # 把记忆存在向量数据库中 memory.save_context({"input": "我喜欢看电影"}, {"output": "不错啊"}) memory.save_context({"input": "我喜欢踢足球"}, {"output": "下次一起啊"}) memory.save_context({"input": "我不喜欢喝咖啡"}, {"output": "ok"}) # 聊到相关话题,检索之前的记忆 print(memory.load_memory_variables({"prompt": "周末做点体育运动?"})["history"])
input: 我喜欢踢足球 output: 下次一起啊
链架构:Chain
以下是摘自官网的描述:
「Chains」允许我们将多个组件组合在一起以创建一个单一的、连贯的应用程序。 例如,我们可以创建一个链,它接受用户输入,使用 PromptTemplate 对其进行格式化,然后将格式化的响应传递给 LLM。 我们可以通过将多个链组合在一起,或者将链与其他组件组合来构建更复杂的链。